The Ultimate Guide to Image Segmentation Annotation Tools
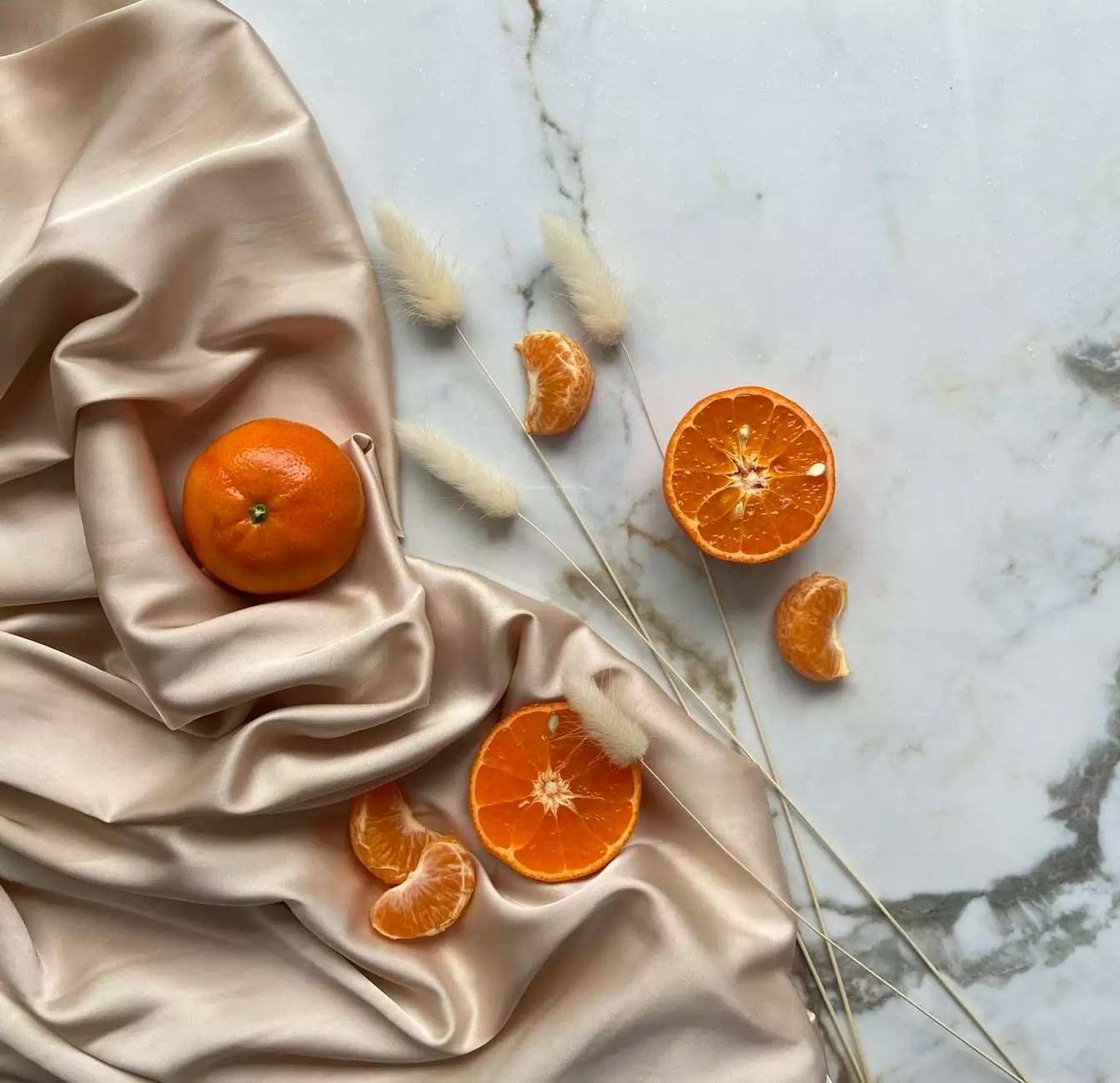
In the evolving landscape of artificial intelligence and machine learning, the importance of data annotation cannot be overstated. It serves as the backbone of AI training processes, impacting the performance of various models significantly. Among the many data annotation techniques, image segmentation is a pivotal aspect, especially when it comes to training models for tasks like object detection, image classification, and scene understanding. In this comprehensive guide, we will delve deep into the world of image segmentation annotation tools, exploring their functionality, importance, and how they can give businesses a competitive edge.
What is Image Segmentation?
Before we dive into the tools, it's crucial to understand what image segmentation entails. In the context of computer vision, image segmentation involves partitioning an image into multiple segments or regions to simplify or change its representation into something more meaningful and easier to analyze.
- Object Detection: Identifying and localizing objects in an image.
- Image Classification: Assigning a label to an entire image.
- Semantic Segmentation: Classifying each pixel in the image into a predefined category.
- Instance Segmentation: Differentiating between separate instances of the same object class.
Image segmentation is a critical process in various applications, including autonomous vehicles, medical imaging, and facial recognition systems. Thus, choosing the right image segmentation annotation tool is paramount for achieving accurate results.
Why Use Image Segmentation Annotation Tools?
The need for specialized tools for image segmentation annotation arises from the complexity of the task. Manual annotation can be tedious and time-consuming, often leading to inaccuracies. Here are several reasons why investing in an image segmentation annotation tool is essential:
- Increased Accuracy: Automated tools significantly reduce human error, leading to more precise annotations.
- Time Efficiency: Speed up the annotation process, allowing teams to focus on analysis rather than data preparation.
- Scalability: Easily handle large datasets which are commonplace in machine learning projects.
- Collaboration: Enhance teamwork by allowing multiple users to access and work on the same project concurrently.
- Cost-Effective: Reduced labor costs associated with manual annotation efforts.
Key Features of Image Segmentation Annotation Tools
When selecting an image segmentation annotation tool, it's important to consider the features that will best serve your project needs. Here are several key features to look for:
1. User-Friendly Interface
A user-friendly interface ensures that both technical and non-technical users can navigate the tool effortlessly. Intuitive design and straightforward navigation reduce the learning curve significantly.
2. Support for Multiple Formats
Your tool should support various image formats (e.g., JPG, PNG, TIFF) to accommodate different types of datasets.
3. Automated Annotation
Some tools come equipped with machine learning algorithms that can automate parts of the annotation process, enhancing productivity. These tools can intelligently suggest segments based on learned patterns.
4. Collaboration Features
Collaboration tools allow for easier team management and project sharing, enabling teams to work together in real-time. Look for features like comments, task assignments, and version control.
5. Robust Export Options
The ability to export annotations in various formats (e.g., COCO, Pascal VOC) is crucial for seamless integration with other AI tools and processes.
Top Image Segmentation Annotation Tools
Investing in the right image segmentation annotation tool can transform your data annotation efforts. Here’s a curated list of some of the top tools available:
1. Keylabs.ai
Keylabs.ai is a powerful platform that specializes in data annotation, providing comprehensive solutions for image segmentation. Its user-friendly interface, combined with advanced features like automation and collaboration tools, makes it ideal for businesses looking to streamline their AI training workflows.
2. Labelbox
Labelbox is well-known for its robust tools for data labeling and management, supporting a wide range of data types, including image segmentation. It offers a blend of manual and automated annotation processes, enhancing efficiency in large datasets.
3. Supervisely
Supervisely combines a modern interface with powerful annotation capabilities. It supports multiple segmentation types and offers integrations with popular machine learning frameworks, making it a popular choice for development teams.
4. VGG Image Annotator (VIA)
VGG Image Annotator is an open-source tool that provides versatile image segmentation capabilities. It’s particularly beneficial for academic and research-focused projects, although its interface might require some technical know-how.
5. RectLabel
RectLabel is tailored for macOS users and provides extensive support for segmenting images and generating compatible datasets for computer vision applications. Its intuitive design simplifies the segmentation process.
Implementing Image Segmentation Annotation Tools in Your Workflow
Integrating an image segmentation annotation tool into your existing workflows can be a game-changer for your data projects. Here’s a step-by-step guide on how to seamlessly implement these tools:
Step 1: Assess Your Data Needs
Identify the nature of your data and determine how much of it requires segmentation. Understanding your requirements will help you choose the right tool and configuration.
Step 2: Choose the Right Tool
Evaluate multiple tools based on features, pricing, and ease of use. Selecting a tool that fits your specific needs is crucial for maximizing productivity.
Step 3: Train Your Team
Once you've chosen your tool, provide adequate training to your team. Familiarity with the tool can drastically improve the speed and quality of annotations.
Step 4: Start Annotating
Begin the annotation process, ensuring that the team adheres to defined guidelines to maintain consistency across the dataset.
Step 5: Review and Optimize
After completing annotations, conduct a thorough review to ensure quality. Look for patterns in errors and refine your processes to enhance accuracy going forward.
Conclusion
In the realm of artificial intelligence, the significance of proper data annotation cannot be overlooked. As we've explored, image segmentation annotation tools are essential for accurately labeling and preparing data for machine learning models. With the right tools, businesses can not only improve the accuracy of their AI models but also significantly enhance their efficiency and productivity.
Embrace the transformation that powerful image segmentation annotation tools can bring. By leveraging these advanced solutions, you’ll position your business at the forefront of innovation, ready to tackle the challenges of tomorrow's technology landscape.
For a deeper insight into how Keylabs.ai can revolutionize your image segmentation projects, visit keylabs.ai today.